Detail Cantuman
Advanced Search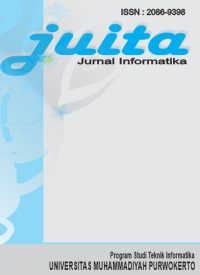
Artikel Jurnal
The Empirical Comparison of Machine Learning Algorithm for the Class Imbalanced Problem in Conformational Epitope Prediction
Abstract
A conformational epitope is a part of a protein-based vaccine. It is challenging to identify using an experiment. A computational model is developed to support identification. However, the imbalance class is one of the constraints to achieving optimal performance on the conformational epitope B cell prediction. In this paper, we compare several conformational epitope B cell prediction models from non-ensemble and ensemble approaches. A sampling method from Random undersampling, SMOTE, and cluster-based undersampling is combined with a decision tree or SVM to build a non-ensemble model. A random forest model and several variants of the bagging method is used to construct the ensemble model. A 10-fold cross-validation method is used to validate the model. The experiment results show that the combination of the cluster-based under-sampling and decision tree outperformed the other sampling method when combined with the non-ensemble and the ensemble method. This study provides a baseline to improve existing models for dealing with the class imbalance in the conformational epitope prediction.
Ketersediaan
JUITA5a-016 | JUITA V9N1 Mei 2021 | Perpustakaan FT UPI YAI | Tersedia |
JUITA5b-016 | JUITA V9N1 Mei 2021 | Perpustakaan FT UPI YAI | Tersedia |
JUITA5c-016 | JUITA V9N1 Mei 2021 | Perpustakaan FT UPI YAI | Tersedia |
Informasi Detil
Judul Seri |
JUITA : Jurnal Informatika
|
---|---|
No. Panggil |
JUITA V9N1 Mei 2021
|
Penerbit | Universitas Muhammadiyah Purwokerto : Purwokerto., 2021 |
Deskripsi Fisik |
hlm : 131-138
|
Bahasa |
English
|
ISBN/ISSN |
2086-9398
|
Klasifikasi |
JUITA
|
Tipe Isi |
-
|
Tipe Media |
-
|
---|---|
Tipe Pembawa |
-
|
Edisi |
Volume 9 Nomor 1 Mei 2021
|
Subyek | |
Info Detil Spesifik |
-
|
Pernyataan Tanggungjawab |
-
|
Versi lain/terkait
Tidak tersedia versi lain