Detail Cantuman
Advanced Search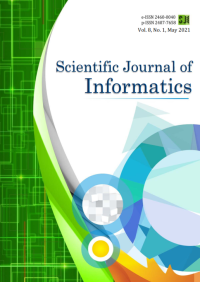
Artikel Jurnal
Deep Learning-based Mobile Tourism Recommender System
Abstract
Purpose: This study developed a deep learning-based mobile travel recommendation system that provides recommendations for local tourist destinations based on users' favorite travel photos. To provide recommendations, use cosine similarity to measure the similarity score between a person's image and a tourism destination gallery through the tag label vector. Label tags are inferred using an image classifier model run from a mobile user device via Tensorflow Lite. There are 40 tag labels that refer to categories, activities and objects of local tourism destinations. Methods: The model is trained using state-of-the-art mobile deep learning architecture EfficientNet-Lite, which is new in the domain of tourism recommender system. Result: This research has conducted several experiments and obtained an average model accuracy of more than 85%, using EfficientNet-Lite as its basic architecture. The implementation of the system as an Android application is proven to provide excellent recommendations with a Mean Absolute Percentage Error (MAPE) of 5.2%. Novelty: A tourism recommendation system is a crucial solution to help tourists discover more diverse tourism destinations. A content-based approach in a recommender system can be an effective way of recommending items because it looks at the user's preference histories. For a cold-start problem in the tourism domain, where rating data or past access may not be found, we can treat the user's past-travel-photos as the histories data. Besides, the use of photos as an input makes the user experience seamless and more effortless.
Ketersediaan
SJI3a-015 | SJI V8N1 May 2021 | Perpustakaan FT UPI YAI | Tersedia |
SJI3b-015 | SJI V8N1 May 2021 | Perpustakaan FT UPI YAI | Tersedia |
Informasi Detil
Judul Seri |
Scientific Journal of Informatics
|
---|---|
No. Panggil |
SJI V8N1 May 2021
|
Penerbit | Universitas Negeri Semarang : Semarang., 2021 |
Deskripsi Fisik |
hlm : 111-118
|
Bahasa |
English
|
ISBN/ISSN |
2407-7658
|
Klasifikasi |
SJI
|
Tipe Isi |
-
|
Tipe Media |
-
|
---|---|
Tipe Pembawa |
-
|
Edisi |
Volume 8 Nomor 1 May 2021
|
Subyek | |
Info Detil Spesifik |
-
|
Pernyataan Tanggungjawab |
-
|
Versi lain/terkait
Tidak tersedia versi lain