Detail Cantuman
Advanced Search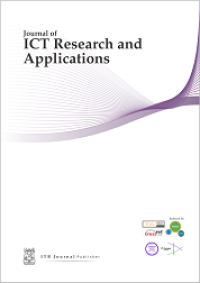
Artikel Jurnal
Unsupervised Detection of Anomalous Sound for Machine Condition Monitoring using Fully Connected U-Net
Abstract
Anomaly detection in the sound from machines is an important task in machine monitoring. An autoencoder architecture based on the reconstruction error using a log-Mel spectrogram feature is a conventional approach for this domain. However, because of the non-stationary nature of some sounds from the target machine, such a conventional approach does not perform well in those circumstances. In this paper, we propose a novel approach regarding the choice of used features and a new auto-encoder architecture. We created the Mixed Feature, which is a mixture of different sound representations, and a new deep learning method called Fully-Connected U-Net, a form of autoencoder architecture. With experiments on the same dataset as the baseline system, using the same architecture for all types of machines, the experimental results showed that our methods outperformed the baseline system in terms of the AUC and pAUC evaluation metrics. The optimized model achieved 83.38% AUC and 64.51% pAUC on average overall machine types on the developed dataset and outperformed the published baseline by 13.43% AUC and 8.13% pAUC.
Ketersediaan
JICTRA4a-003 | JICTRA V15N1 June 2021 | Perpustakaan FT UPI YAI | Tersedia |
JICTRA4b-003 | JICTRA V15N1 June 2021 | Perpustakaan FT UPI YAI | Tersedia |
JICTRA4c-003 | JICTRA V15N1 June 2021 | Perpustakaan FT UPI YAI | Tersedia |
Informasi Detil
Judul Seri |
Journal of ICT Research and Application
|
---|---|
No. Panggil |
JICTRA V15N1 June 2021
|
Penerbit | ITB Journal Publisher : Bandung., 2021 |
Deskripsi Fisik |
hlm : 41-55
|
Bahasa |
English
|
ISBN/ISSN |
2337-5787
|
Klasifikasi |
JICTRA
|
Tipe Isi |
-
|
Tipe Media |
-
|
---|---|
Tipe Pembawa |
-
|
Edisi |
Volume 15 Nomor 1 June 2021
|
Subyek | |
Info Detil Spesifik |
-
|
Pernyataan Tanggungjawab |
-
|
Versi lain/terkait
Tidak tersedia versi lain