Detail Cantuman
Advanced Search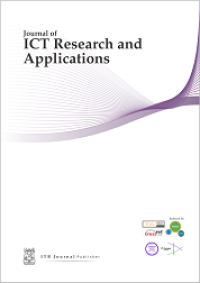
Artikel Jurnal
Machine-Learning Classifiers for Malware Detection Using Data Features
Abstract
The spread of ransomware has risen exponentially over the past decade, causing huge financial damage to multiple organizations. Various anti-ransomware firms have suggested methods for preventing malware threats. The growing pace, scale and sophistication of malware provide the anti-malware industry with more challenges. Recent literature indicates that academics and anti-virus organizations have begun to use artificial learning as well as fundamental modeling techniques for the research and identification of malware. Orthodox signature-based anti-virus programs struggle to identify unfamiliar malware and track new forms of malware. In this study, a malware evaluation framework focused on machine learning was adopted that consists of several modules: dataset compiling in two separate classes (malicious and benign software), file disassembly, data processing, decision making, and updated malware identification. The data processing module uses grey images, functions for importing and Opcode n-gram to remove malware functionality. The decision making module detects malware and recognizes suspected malware. Different classifiers were considered in the research methodology for the detection and classification of malware. Its effectiveness was validated on the basis of the accuracy of the complete process.
Ketersediaan
JICTRA6-005 | JICTRA V15N3 December 2021 | Perpustakaan FT UPI YAI | Tersedia |
Informasi Detil
Judul Seri |
Journal of ICT Research and Application
|
---|---|
No. Panggil |
JICTRA V15N3 December 2021
|
Penerbit | ITB Journal Publisher : Bandung., 2021 |
Deskripsi Fisik |
hlm : 265-290
|
Bahasa |
English
|
ISBN/ISSN |
2337-5787
|
Klasifikasi |
JICTRA
|
Tipe Isi |
-
|
Tipe Media |
-
|
---|---|
Tipe Pembawa |
-
|
Edisi |
Volume 15 Nomor 3 December 2021
|
Subyek | |
Info Detil Spesifik |
-
|
Pernyataan Tanggungjawab |
-
|
Versi lain/terkait
Tidak tersedia versi lain