Detail Cantuman
Advanced Search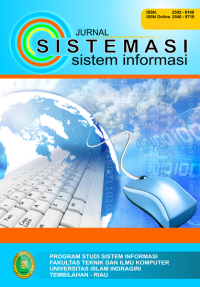
Artikel Jurnal
Comparative Analysis of Data Mining Classification Algorithm Performance for Searching Prospective Student Interests
Abstract
Admission of new students is an activity that’s always carried out by every university in the new academic year. The decline in the number of registrants every year is an obstacle for AMIK HASS in new student admissions, efforts are needed to process the existing data on new student admissions. Data mining applications use classification algorithms that aim to make predictions. The algorithms used are Nave Bayes (NB), Decision Tree J48 (J48), K-Nearest Neighbor (K-NN), Random Forest (RF), and Support Vector Machine (SVM). Algorithm testing to analyze the performance of each algorithm uses WEKA. The data set used in this study is the search for potential and interest of prospective new students as many as 5,934 records. The tests carried out on the five algorithms use the test percentage split mode, which is 70% for training data and 30% for test data. The highest accuracy rate on J48 is 90.34% followed by RF at 89.04%, SVM at 88.43%, K-NN at 87.53%, NB at 87.25%. J48 is the best algorithm for testing data sets with the lowest prediction error rate of 0.26. The J48 classification algorithm has explicit rules for the classification and handling of heterogeneous data by having 255 rules.
Ketersediaan
SISTEMASI6a-002 | SISTEMASI V11N2 Mei 2022 | Perpustakaan FT UPI YAI | Tersedia |
SISTEMASI6b-002 | SISTEMASI V11N2 Mei 2022 | Perpustakaan FT UPI YAI | Tersedia |
Informasi Detil
Judul Seri |
SISTEMASI : Jurnal Sistem Informasi
|
---|---|
No. Panggil |
SISTEMASI V11N2 Mei 2022
|
Penerbit | Universitas Islam Indragiri : Riau., 2022 |
Deskripsi Fisik |
hlm : 271-290
|
Bahasa |
Indonesia
|
ISBN/ISSN |
2302-8149
|
Klasifikasi |
SISTEMASI
|
Tipe Isi |
-
|
Tipe Media |
-
|
---|---|
Tipe Pembawa |
-
|
Edisi |
Volume 11 Nomor 2 Mei 2022
|
Subyek | |
Info Detil Spesifik |
-
|
Pernyataan Tanggungjawab |
-
|
Versi lain/terkait
Tidak tersedia versi lain