Detail Cantuman
Advanced Search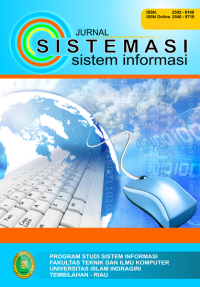
Artikel Jurnal
Hyperparameter Tuning on Classification Algorithm with Grid Search
Abstract
Currently, machine learning algorithms continue to be developed to perform optimization with various methods to produce the best-performing model. In Supervised learning or classification, most of the algorithms have hyperparameters. Tuning hyperparameter is an architecture of deep learning to improve the performance of predictive models. One of the popular hyperparameter methodologies is Grid Search. Grid Search using Cross Validation provides convenience in testing each model parameter without having to do manual validation one by one. In this study, we will use a method in hyperparameter optimization, namely Grid Search. The purpose of this study is to find out the best optimization of hyperparameters against 7 machine learning classification algorithms. Validation of experimental results using the Mean Cross Validation. The experimental results show that the XGBoost model gets the best value while the Decision tree has the lowest value.
Ketersediaan
SISTEMASI6a-010 | SISTEMASI V11N2 Mei 2022 | Perpustakaan FT UPI YAI | Tersedia |
SISTEMASI6b-010 | SISTEMASI V11N2 Mei 2022 | Perpustakaan FT UPI YAI | Tersedia |
Informasi Detil
Judul Seri |
SISTEMASI : Jurnal Sistem Informasi
|
---|---|
No. Panggil |
SISTEMASI V11N2 Mei 2022
|
Penerbit | Universitas Islam Indragiri : Riau., 2022 |
Deskripsi Fisik |
hlm : 391-401
|
Bahasa |
Indonesia
|
ISBN/ISSN |
2302-8149
|
Klasifikasi |
SISTEMASI
|
Tipe Isi |
-
|
Tipe Media |
-
|
---|---|
Tipe Pembawa |
-
|
Edisi |
Volume 11 Nomor 2 Mei 2022
|
Subyek | |
Info Detil Spesifik |
-
|
Pernyataan Tanggungjawab |
-
|
Versi lain/terkait
Tidak tersedia versi lain