Detail Cantuman
Advanced Search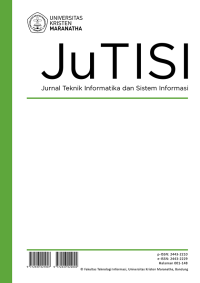
Artikel Jurnal
Pengaruh Metode Penyeimbangan Kelas Terhadap Tingkat Akurasi Analisis Sentimen pada Tweets Berbahasa Indonesia
Abstract
Nowadays internet access is getting easier to get. Because of the ease of access to the internet, almost all internet users have social media. Social media is widely used by users to call out their opinions or even to make complaints about a matter and also discuss a topic with other social media users. From many existing social media, one that is popularly used for that activity is Twitter. Sentiment analysis on Twitter has become possible because of the activities of these Twitter users. In this research, the authors explore sentiment analysis with bag-of-words and Term Frequency Inverse Document Frequency (TF-IDF) features extraction based on tweets from Indonesian Twitter users. The data obtained is in imbalanced condition, so that it requires a method to overcome them. The method for overcoming imbalanced dataset uses a resampling approach which combines over and under sampling strategies. The results of sentiment analysis accuracies with Naïve Bayes and neural networks before and after input data resampling are also compared. Naïve Bayes methods that will be used are Multinomial Naïve Bayes and Complement Naïve Bayes, while the Neural Network architecture that will be used as a comparison are Recurrent Neural Networks, Long Short-Term Memory, Gated Recurrent Units, Convolutional Neural Networks, and a combination of Convolutional Neural Networks and Long Short-Term Memory. Our experiments show the following harmonic scores (F1) of the sentiment analysis models: the Multinomial Naïve Bayes F1 score is 55.48, Complement Naïve Bayes is 51.33, Recurrent Neural Network is 75.70, Long Short-Term Memory is 78.36, Gated Recurrent Unit is 77.96, Convolutional Neural Network is 76.12, and finally the combination of Convolutional Neural Networks and Long Short-Term Memory achieves 81.14.
Ketersediaan
JUTISI2-022 | JUTISI V6N2 Agustus 2020 | Perpustakaan FT UPI YAI | Tersedia |
Informasi Detil
Judul Seri |
JUTISI : Jurnal Teknik Informatika dan Sistem Informasi
|
---|---|
No. Panggil |
JUTISI V6N2 Agustus 2020
|
Penerbit | Maranatha University Press : Bandung., 2020 |
Deskripsi Fisik |
hlm : 400-413
|
Bahasa |
Indonesia
|
ISBN/ISSN |
2443-2210
|
Klasifikasi |
JUTISI
|
Tipe Isi |
-
|
Tipe Media |
-
|
---|---|
Tipe Pembawa |
-
|
Edisi |
Volume 6 Nomor 2 Agustus 2020
|
Subyek | |
Info Detil Spesifik |
-
|
Pernyataan Tanggungjawab |
-
|
Versi lain/terkait
Tidak tersedia versi lain